EVALUATION OF NECK FATIGUE CAUSED BY WEARING HELMET IN MOTION: AN ELECTROMYOGRAPHY STUDY
Abstract
The increased weight of multifunction and intelligent helmet systems can cause an exacerbation of the neck workload. Further, increased weight of the helmet can reduce the wearing comfort, and prolonged use may cause neck fatigue and even neck injury. This study evaluates the fatigue of neck muscles caused by wearing a helmet in motion using electromyography (EMG) measurement. Additionally, a comprehensive evaluation model for neck fatigue was established effectively. Twenty healthy subjects were selected to run for 10min continuously in each task by wearing two different helmets, respectively, during which the EMG signals of different neck muscles and subjective scores were recorded and analyzed. Two-way repeated measures analysis of variance (ANOVA) and Pearson correlation analysis were used to obtain indicators of neck fatigue, based on which the support vector machine (SVM) was used to construct a classification model of neck fatigue. By using integral EMG (IEMG) and root mean square (RMS) for assessing the neck injury in sternocleidomastoideus and trapezius muscles, it was observed that the mean power frequency (MPF) and median frequency (MF) in trapezius and splenius capitis could be used as effective evaluation indicators of neck fatigue. The EMG activity in trapezius was the highly sensitivity to fatigue when wearing helmets in motion. The classifier for distinguishing three different neck fatigue levels was 91.67% accurate. This study demonstrates the feasibility of an EMG-based method to evaluate the neck fatigue caused by wearing a helmet in motion, and also provides an evaluation method for the optimal design of helmets in the future.
1. Introduction
With the rapid development of high technology, helmet is no longer a simple protection tool, but gradually has become an intelligent helmet system integrating multiple functions.1,2,3 In particular, with the penetration of artificial intelligence technology into the military field, the future soldier system also shows a trend of intelligent development. The wearing of intelligent helmets by soldiers is also regarded as a man–machine joint combat system, which is likely to change the combat mode of modern war. Such “future soldiers” integrating the land, air and machine in science fiction may enter the war stage.4,5 More and more modules such as helmet-mounted displays, radio communication systems, visual tracking and targeting systems, night vision devices, night vision goggles, and oxygen masks are being installed in the helmet, in order to improve the situational awareness and control ability of the soldier. However, at the same time, it is accompanied by new problems.6,7 The weight of the helmet gets increased in the process, and the load on the human body gradually increases.8,9 In particular, the human neck is composed of neck muscles, vertebrae and ligaments with small structures and weak carrying capacity, and soldiers are often accompanied by fierce tactical actions in the course of combat. In this case, as the mass of the helmet increases, the center of mass of the helmet deviates from the center of mass of the head. In order to balance this torque, a series of neck muscles contract or expand. As a result, the uneven distribution of muscle force in the head and neck causes muscle fatigue or damage, which reduces the comfort of wearing the helmet and can lead to cervical spondylosis in several cases.10,11,12 Therefore, the intellectualization of the helmet also puts forward new requirements for the ergonomic design of the helmet.
Previous scholars paid more attention to the stiffness and strength of the helmet shell to avoid head injury caused by collision.13,14,15,16,17 The influence of helmet weight on the neck began from the Swedish Air Force, and then scholars at home and abroad have carried out a lot of theoretical analysis and experimental research. Liu et al.18 found that helmet weight was one of the important causes of pilots’ neck symptoms from a study of a large sample size of pilots. As a result, the helmet weight has become a breakthrough to solve pilots’ neck health problems. Thuresson19 found that the heavy counterweight of the helmet-mounted display system (HMDS) and the continuous uncomfortable working posture were the main causes of neck injury. Researchers have carried out a large number of studies on helmet comfort and the impact of helmet weight on neck fatigue, mostly focusing on subjective evaluation, biomechanical method, mathematical simulation model, and biochemical methods. Jia et al.10 used the musculoskeletal model established by Anybody to study the effect of helmet mass and center of mass position on neck muscles, and the results provided a qualitative and quantitative reference for the design and use of helmets. Wu et al.20 conducted experiments using helmets that were worn by hybrid dummies and volunteers, and measured the biomechanical effects of helmets on the human body under different accelerations. Yang et al.21 established a “helmet–head–neck” musculoskeletal model and analyzed the effects of helmet mass, center of mass position and jaw strap tightening force on the movement characteristics of head and neck muscle groups by using reverse dynamics. Other studies assessed the muscle fatigue by measuring the content of lactic acid in the blood.22,23 All these studies have important guiding significance considering the helmet design and comfort evaluation, but there are still some shortcomings in this work, such as the subjective evaluation method is greatly affected by individual differences, and the helmet-neck model based on multi-rigid body dynamics is too simplified and ignores the force of muscles. The measurement of lactic acid content in blood is invasive and unacceptable. In recent years, with the improvement of physiological signal analysis technology, the application and analysis of electromyography (EMG) has attracted extensive attention from scholars at home and abroad.24,25,26 EMG is a neuromuscular activity electrical signal that is collected and recorded by electrodes on the surface of human skeletal muscle and superimposed by muscle fiber action potentials in time and space to represent muscle activity and function status, which is closely related to muscle fatigue degree and contractile force. Studies have proved that EMG generated by muscle force can reflect local fatigue characteristics, so it is reasonable for its application in the detection and evaluation of local neck muscle activity level and fatigue state.27,28,29,30,31,32
This study mainly focuses on the EMG study of neck fatigue caused by wearing a helmet in motion. Along with the subjective evaluation, multiple EMG characteristic parameters in different neck muscles were extracted and analyzed. The sensitivity of these parameters for evaluating neck fatigue was investigated to identify objective and effective indicators. Based on these parameters, principal component analysis (PCA) was used to reduce the dimension of the data and a new set of samples was formed as the model input. Considering the insufficient samples, support vector machine (SVM) was proposed and used to establish an effective model for evaluating neck fatigue.
2. Materials and Methods
2.1. Subjects
Twenty healthy young males (age=25.3±4.2 years; height=170.5±6.0cm; weight=67.3±7.15kg) were selected and participated in the study. All had no neck muscle disease, which was mainly determined by the subject’s chief complaint and the examination and diagnosis of orthopedic doctors. All subjects were in good health and did not engage in any form of vigorous exercise within 24h before the experiment. All were informed of the purpose and content of the experiment and given written informed consent to participate in the experiment, and they were compensated with $40 after their participation.
2.2. Experimental design
This study designed an experiment on the influence of wearing a helmet on neck muscles during continuous running. In order to compare their differences and consistency, two helmets with different mass properties were selected, as shown in Table 1. According to sports science and human biological anatomy, the muscles involved in neck activities caused by helmet-wearing in motion were analyzed, and the sternocleidomastoideus, trapezius, and splenius capitis were initially selected as EMG signal collection sites. The experiment adopted a 2×3×5 within-subjects design (helmet×muscle×time), and the independent variables included intra- (time) and intergroup variables (helmet), while the dependent variables were EMG parameters of different neck muscles in time and frequency domain: integral EMG (IEMG), root mean square (RMS), mean power frequency (MPF) and median frequency (MF).
Center-of-gravity position | |||
---|---|---|---|
Name | Mass (kg) | X/m | Z/m |
Helmet I | 4.16 | 0.027 | 0.01 |
Helmet II | 5.82 | 0.026 | 0.02 |
The experiment was carried out in an electrically shielded and soundproof room. The ambient temperature was controlled at 23–24∘C and the humidity was controlled at 40–50%. Figure 1 shows the experiment procedure, which consisted of two running tasks wearing different helmets. Each subject performed the two tasks, respectively, and they were required to wear helmets firmly without sloshing and run on a treadmill for 10min with the treadmill speed setting at 6km/h. EMG signals were recorded during the task, and meanwhile, subjects were asked to give their comprehensive fatigue scores and local muscle fatigue scores in oral forms, respectively, according to Tables 2 and 3 every 2min. To avoid the fatigue effect induced by continuous running tasks on experimental results, subjects were required to take a break for 15–20min after completing one running task, and then proceeded with the other task. In addition, the subjects were asked to randomly select two helmets to carry out the task in sequence. The experiment scene is shown in Fig. 2.
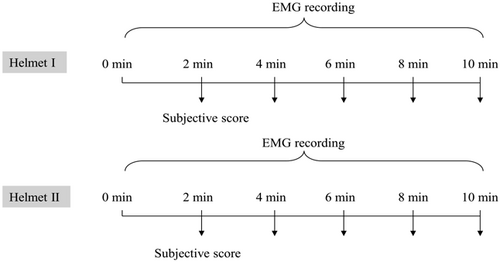
Fig. 1. Experimental setup.
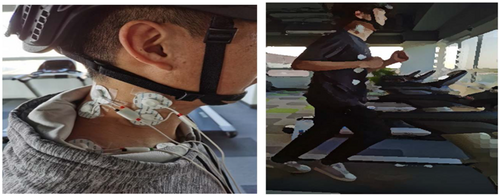
Fig. 2. Experimental scene.
Fatigue level | Descriptions | Score |
---|---|---|
Relaxed | No fatigue, no effort | 1 |
Slightly relaxed | Relaxed, but feel different from the beginning | 2 |
Slightly tired | Slight muscle fatigue, slightly difficult to operate | 3 |
Tired | Mild acid distension with a desire to stretch the muscles, distracted but still able to persist | 4 |
Very tired | Muscles ache, suppress muscle stretch with difficulties, breathing quickens, and a decreased willingness to persist | 5 |
Extremely tired | Increased muscle ache, significantly faster breathing, no longer willingness to persist | 6 |
Exhausted | Obvious muscle aches, shortness of breath, can no longer persist | 7 |
Fatigue level | Descriptions | Score |
---|---|---|
Relaxed | No effect or symptom | 1 |
Slightly relaxed | Slight muscle tightness | 2 |
Mild soreness | Slight muscle soreness, adjusting posture to relieve | 3 |
Moderate soreness | Muscle soreness and stiffness, slightly interfering with muscle contraction | 4 |
Severe soreness | Muscles ache and stiffness, intensively interfering with muscle contraction | 5 |
2.3. Recording and processing of the EMG signal
EMG signals were amplified and recorded with EMG channels of a multi-channel physiological signal recording and analysis system (Biosignals series, PLUX). The signals were digitalized continuously with a sampling rate of 1000Hz with an 8–500Hz band-pass filter and 50Hz notched, and input impedance ≥10GΩ, gain 1000, common-mode rejection ratio (CMRR) 100dB, A/D conversion (12-bit).
Since there were many artifacts in the raw EMG signals, such as 50Hz frequency interference and pseudo-errors caused by poor electrodes’ contact or body movement. A Butterworth filter was designed to achieve 20–150Hz band-pass and 50Hz band-stop filtering in this study. Two methods, those were, time domain analysis and frequency domain analysis were used to process the preprocessed EMG signals by MATLAB. The EMG time domain parameters (IEMG, RMS) and frequency domain parameters (MPF, MF) were chosen as the assumed indicators of neck fatigue for the later analysis in this study. The frequency domain parameters were obtained based on discrete Fourier transformation (DFT) of the EMG signals.
3. Results
3.1. Subjective results
Figure 3 shows the results of the mean comprehensive fatigue scores and three local muscle fatigue scores over time for the subjects wearing different helmets during running. It can be seen that the comprehensive scores and three local muscle fatigue scores showed significant increasing trends with time. Meanwhile, the fatigue degree for subjects wearing helmet II was greater than that wearing helmet I.
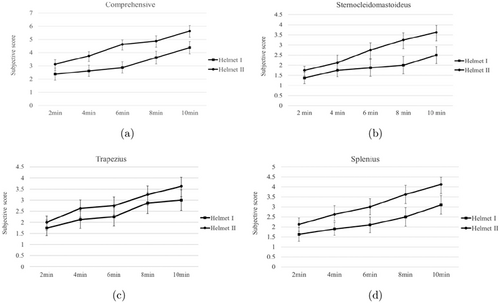
Fig. 3. Subjective evaluation results: (a) comprehensive; (b) sternocleidomastoideus; (c) trapezius; (d) splenius capitis.
3.2. EMG results
The artifact-removed EMG signals obtained from each 10min running task were divided into five 2-min sections evenly over time, based on which the four characteristic parameters: IEMG, RMS, MPF, MF of each 2-min section were calculated. As a result, 2×3×5 (Helmet×Muscle×Time) of EMG parameters set (IEMG, RMS, MPF, MF) were obtained.
First, the 3σ criterion was used to eliminate the singular data, and then two-way repeated measures analysis of variance (ANOVA) (intra-variable: time; intergroup variable: helmet) was used to analyze each EMG parameter in each muscle. The difference analysis results of intra-variable and intergroup variables are shown in Tables 4 and 5, respectively. Taking the trapezius as an example, the results of four EMG parameters over time for the subjects wearing different helmets during running can be found in Fig. 4. According to Table 4 and the changing trends of four EMG parameters shown in Fig. 4, it can be observed that IEMG and RMS increased significantly in sternocleidomastoideus and trapezius (all p<0.05) with an increase in the running duration, while MPF and MF decreased significantly in trapezius and splenius capitis (all p<0.05). As shown in Table 5, the difference analysis results of EMG parameters in helmet factor proved that the IEMG and RMS in trapezius for subjects wearing helmet II were significantly greater than those wearing helmet I (all p<0.05), while MPF and MF for subjects wearing helmet II were significantly lower than that wearing helmet I (all p<0.05).
Muscle | IEMG | RMS | MPF | MF |
---|---|---|---|---|
Sternocleidomastoideus | p<0.05 | p<0.05 | / | / |
Trapezius | p<0.05 | p<0.05 | p<0.05 | p<0.05 |
Splenius capitis | / | / | p<0.05 | p<0.05 |
Muscle | IEMG | RMS | MPF | MF |
---|---|---|---|---|
Sternocleidomastoideus | / | / | / | / |
Trapezius | p<0.05 | p<0.05 | p<0.05 | p<0.05 |
Splenius capitis | / | / | / | / |
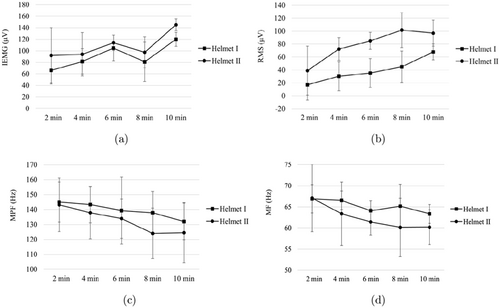
Fig. 4. Changes in EMG parameters with time in trapezius: (a) IEMG; (b) RMS; (c) MPF; (d) MF.
In conclusion, IEMG and RMS in sternocleidomastoideus, IEMG, RMS, MPF and MF in trapezius, and MPF and MF in splenius capitis can be used as potential evaluation indicators of neck fatigue. Furthermore, the EMG activities in the trapezius offered the highest sensitivity to fatigue when wearing a helmet in motion compared with those in the other two neck muscles.
3.3. Subjective and objective consistency analysis
In order to verify the validity of the EMG parameters as evaluation indicators of neck fatigue, Pearson correlation analysis was used to conduct the subjective and objective consistency analysis between EMG characteristic parameters and subjective fatigue scores in trapezius as an example. As shown in Fig. 5, it can be seen that IEMG and RMS were strongly positively correlated with the subjective fatigue scores (all |r|>0.6, p<0.05), while MPF and MF were strongly negatively correlated with the subjective fatigue scores (all |r|>0.6, p<0.05), which further verified the previous conclusion.
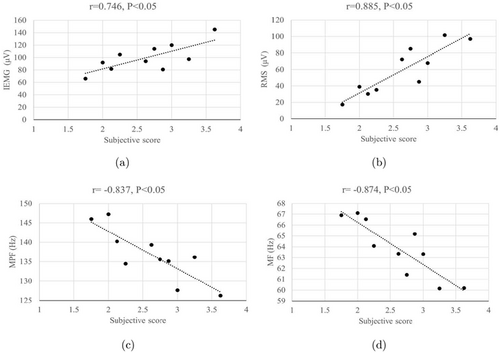
Fig. 5. Pearson correlation analysis results: (a) IEMG; (b) RMS; (c) MPF; (d) MF.
3.4. Fatigue classification
Due to the individual differences, it was difficult to establish a quantitative fatigue model with traditional statistical methods. The evaluation of neck fatigue was regarded as a pattern classification problem in this study, which was trained according to the sample sets generated under different fatigue states. Subsequently, the trained classifier was used to further identify the fatigue category of the test samples. Considering the insufficient samples, SVM was proposed and used in this study, as it is a classification algorithm based on the structural risk minimization (SRM) principle.33,34,35 Initially, IEMG and RMS in sternocleidomastoideus, IEMG, RMS, IEMG and RMS in trapezius, and IEMG and RMS in splenius capitis were selected and constituted into a multidimensional original sample. After standardizing the original sample to unified dimension, PCA was used to extract the principal elements and finish the multi-features fusion, and a new sample set were developed as the input of SVM. The sample sets were classified into three groups with different fatigue levels according to corresponding comprehensive fatigue scores. The samples of the comprehensive fatigue scores between 1 and 2 were labeled as level-1 group, 2–4 as level-2 group, and 4–7 as level-3 group (level-1, level-2, and level-3 were expressed by 1, 2, 3, respectively). 75% data of each group was chosen randomly and constituted as the training sample set, while the rest as the test sample set. The LIBSVM ToolBox of MATLAB was used to complete the classification for three levels of fatigue. Radial basis kernel were chosen as the kernel function in this study, and the grid search algorithm was used for parameter optimization (c, g), while the K-fold cross-validation (K-CV) was used for cross-validation, among which the range of values for log2(c) and log2(g) were [−4, 4], K=3. The parameter searching results of the optimization process (contour map and 3D view) are shown in Fig. 6, in which, the loss function parameter c=2, regularization parameter g=0.25, and accuracy=92.71%. Based on these parameters, the SVM classifier was built and verified, and the classification testing results (Predicted_ACC=91.67%) and confusion matrix for the test set are shown in Fig. 7. The results indicate that the established classifier based on the fusion of EMG parameters in this study exhibited a good performance.
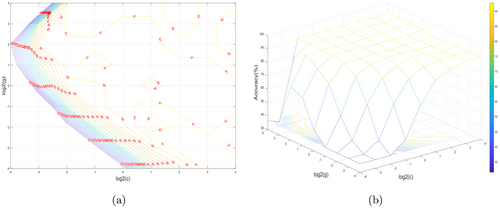
Fig. 6. Optimization of radial basis kernel function parameters: (a) contour map; (b) 3D view.
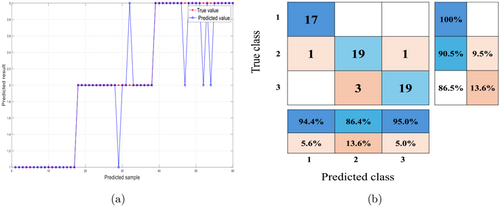
Fig. 7. SVM classification testing result: (a) predicted result comparison of test set; (b) confusion matrix for test set.
4. Discussion
In this study, the evaluation methods of neck fatigue with helmet wearing in motion were studied. Subjective evaluation was taken as an auxiliary measurement, and various indicators were calculated and verified from the perspective of EMG in the time domain and frequency domain. The EMG activities in different neck muscles were discussed and the characteristic parameters related to neck fatigue were extracted. Based on these characteristic parameters, the SVM classifier was constructed to classify neck fatigue levels.
The subjective evaluation results indicated that the fatigue of comprehensive and local muscles increased along with the running time, and helmet weight also exacerbated the fatigue degree. The human head is in a slightly bent state in normal posture, and the neck muscles work together to maintain the balance of the head posture and motion when they wear helmets to run. The EMG activities in sternocleidomastoideus, trapezius, and splenius capitis were studied in this study, and it was found that the IEMG and RMS were increased significantly in sternocleidomastoideus and trapezius, while MPF and MF decreased significantly in trapezius and splenius capitis. In previous studies, IEMG and RMS have been proven to reflect the amplitude of EMG signals in the time domain, which are positively correlated with muscle force.36 With the accumulation of fatigue effect during running with helmet wearing, the number of muscle fiber motor units recruited by the nervous system increases, in order to maintain the required force for movement, thereby increasing the amplitude of EMG.37 The significant decrease of MPF and MF was because of the action potential conduction speed of the fiber motor unit that decreased with the fatigue accumulation. Further, the spectrum of the EMG signal shifted to the left, that is, the power spectral density of the low-frequency band increased, and the power spectral density of the high-frequency band decreased, which resulted in a decrease of MPF and MF in the trapezius and splenius capitis.38,39,40 The results show that the EMG activities in sternocleidomastoideus, trapezius, and splenius capitis can be used as effective measurements to evaluate neck fatigue. In addition, it was found that trapezius was the most sensitive to neck fatigue, so it is more susceptible to damage under the condition of running with a helmet for a prolonged duration.
5. Conclusion
Our findings demonstrate that EMG could be effectively used to evaluate the different levels of neck fatigue due to wearing a helmet in movement. IEMG, RMS, MPF and MF in different neck muscles proved to be effective indicators of neck fatigue. Based on these indicators, the method of SVM based on PCA was used to establish an effective classification model with an accuracy of 91.67% for three classes. This study demonstrated that the proposed algorithm could be applied to neck fatigue evaluation.
Although valuable findings were obtained, there are still some limitations to this study. First, the sample size was too small that the final results might be affected by the individual differences. Second, in addition to the helmet weight, other parameters like the position of the centroid position affecting neck fatigue need to be evaluated, and the mechanisms still need to be explored based on this research. Moreover, EMG measurement can only reflect the activity characteristics of surface muscles of neck fatigue, and it is difficult to evaluate the muscles’ strength and the influence of helmet parameters on them comprehensively and accurately. Therefore, future studies should include other measurements, such as musculoskeletal model construction, combined with EMG evaluation.
Acknowledgments
This research was funded by the National Natural Science Fund (82102176) named “Research on VR Evoked Emotion Recognition Method Based on Multimodal Physiological Signal Fusion”.
ORCID
Xiaoli Fan https://orcid.org/0000-0001-8731-7454
Feng Wu https://orcid.org/0009-0006-6303-8569
Andong Zhao https://orcid.org/0009-0007-0964-8152
Hua Ge https://orcid.org/0000-0003-3098-3258
Heqing Liu https://orcid.org/0009-0008-8712-4503
Yanpeng Zhao https://orcid.org/0009-0007-3508-7109